Unsupervised learning, a subset of machine learning, has the potential to revolutionize education by uncovering hidden patterns and relationships in vast amounts of data without the need for labeled datasets. In an educational context, it can be applied to personalize learning experiences, identify student groups with similar learning needs, and enhance the overall effectiveness of teaching methods. This article explores the benefits of unsupervised learning in education, delving into key techniques, practical applications, and real-world case studies. By understanding the challenges and future trends in this area, educators and institutions can unlock the full potential of unsupervised learning, paving the way for more adaptive and data-driv
Join electrapk.com for a detailed examination of this topic.
1. Introduction to Unsupervised Learning
Unsupervised learning is a type of machine learning where algorithms explore data without explicit guidance. Unlike supervised learning, which relies on labeled datasets, unsupervised learning allows the model to uncover patterns, clusters, and relationships within the data autonomously. This ability makes it especially valuable for extracting knowledge from intricate, uncategorized data.
Unsupervised learning holds transformative potential in education. It enables educators to gain valuable insights into student behavior, learning preferences, and performance trends without requiring explicit labels or guidance. By clustering students with similar learning styles or analyzing vast amounts of educational data, educators can tailor their teaching strategies to better suit individual needs and improve learning outcomes. Moreover, unsupervised learning can assist in curriculum development by pinpointing gaps in content or identifying areas where students consistently struggle.
This section introduces the concept of unsupervised learning, laying the groundwork for exploring its applications and benefits within the realm of education. Understanding the principles of unsupervised learning will empower educators and administrators to recognize its potential for revolutionizing traditional educational practices, paving the way for more personalized and efficient learning systems.
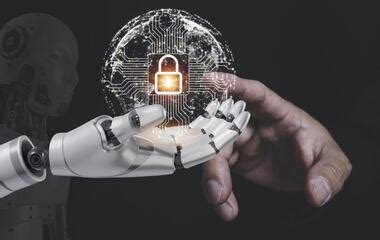
2. Benefits of Unsupervised Learning in Education
Unsupervised learning presents significant advantages for education by offering valuable insights that can be used to personalize learning experiences. One of its key strengths lies in its ability to identify patterns within student performance data. By analyzing these patterns, educators can group students with similar learning styles and challenges. This facilitates more focused interventions and tailored teaching strategies, ultimately enhancing student engagement and academic achievement.
Additionally, unsupervised learning can enhance curriculum development by analyzing vast amounts of educational data to pinpoint gaps or areas where students collectively struggle. This data-driven approach helps educators refine course content, ensuring it meets the diverse needs of learners.
Furthermore, unsupervised learning supports early identification of at-risk students by detecting subtle trends in behavior and performance that might otherwise go unnoticed. By proactively addressing these issues, educators can provide timely support, fostering better educational outcomes. Overall, unsupervised learning empowers educators with deeper insights, leading to more effective and adaptive teaching methods.
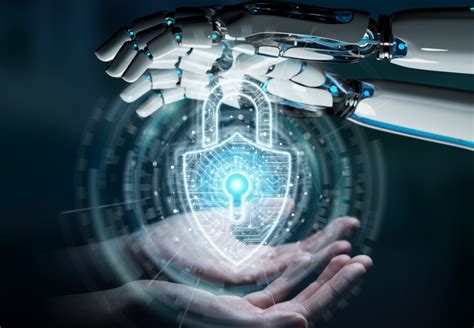
3. Key Unsupervised Learning Techniques
Unsupervised learning plays a vital role in the analysis and interpretation of educational data. Several techniques contribute to this process, with clustering, association, and dimensionality reduction being prominent examples.
Clustering, a commonly used technique in education, involves grouping students, assignments, or learning materials based on shared characteristics. This practice allows for tailored instruction by, for example, forming groups of students with similar learning styles or performance levels. Clustering is especially beneficial in large classrooms where individualized attention may be difficult to provide.
Association is a powerful technique that helps us understand relationships between different variables. In education, it can be used to identify patterns in student behavior, like the resources they use most often or the mistakes they commonly make. This understanding allows educators to see how different parts of the learning environment interact. This knowledge then empowers educators to improve teaching methods and optimize the use of resources.
Dimensionality reduction simplifies complex datasets by reducing the number of variables, preserving essential information. In education, this technique can streamline large volumes of data, such as student performance metrics, making it easier for educators to analyze and draw meaningful conclusions.
By effectively employing clustering, association, and dimensionality reduction techniques, educators can unlock valuable insights from raw educational data. This process allows them to identify hidden patterns, refine teaching methods, and ultimately create more effective and personalized learning environments.
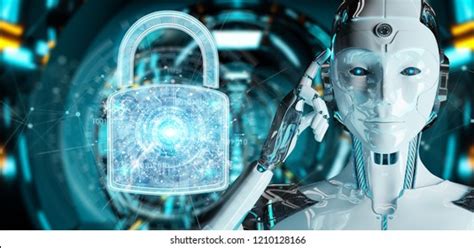
4. Applications in Educational Settings
Unsupervised learning has diverse applications in educational settings, enhancing both teaching and administrative functions. One prominent application is personalized learning. By clustering students based on their learning styles and performance, educators can tailor instruction to meet individual needs, making lessons more engaging and effective.
Another application is in curriculum design. Unsupervised learning can analyze large datasets of student performance to identify content areas where students struggle. This allows educators to refine and adapt the curriculum to address these challenges, ensuring that it remains relevant and effective.
Additionally, unsupervised learning plays a vital role in resource optimization. By identifying patterns in student resource usage, schools can allocate materials and support services more efficiently, ensuring that all students have access to what they need.
Finally, unsupervised learning aids in early intervention by detecting at-risk students based on subtle trends in their academic behavior. This proactive approach allows educators to provide timely support, helping students sta
5. Case Studies and Examples
Several case studies illustrate the impactful use of unsupervised learning in education. One notable example is a university that used clustering techniques to analyze student performance data. By grouping students based on their academic progress, the university identified patterns of underperformance in specific cohorts. This insight allowed them to implement targeted support programs, resulting in improved student retention and graduation rates.
In another example, a school district employed unsupervised learning to optimize resource allocation. By analyzing patterns in student behavior and resource usage, the district identified underutilized materials and redeployed them where they were most needed. This led to more effective use of educational resources and a better learning experience for students.
An online learning platform enhanced personalization by employing unsupervised learning to recommend courses. By grouping students based on their interactions with course materials, the platform could suggest courses tailored to individual learning preferences. This resulted in greater student engagement and improved course completion rates.
These case studies showcase the adaptability and effectiveness of unsupervised learning across different educational settings. Whether its purpose is to bolster student support, streamline resources, or tailor learning experiences, unsupervised learning delivers valuable insights that lead to improved educational results.
6. Challenges and Limitations
Despite its potential, unsupervised learning in education faces several challenges and limitations. One primary challenge is the complexity of the data. Educational data is often unstructured and noisy, making it difficult for unsupervised algorithms to identify meaningful patterns without extensive preprocessing. This can lead to inaccurate or misleading results if not handled carefully.
Another limitation is the interpretability of the models. Unsupervised learning algorithms can produce complex outputs that are challenging for educators and administrators to understand and apply effectively. This lack of transparency can hinder the implementation of insights derived from the data.
Additionally, unsupervised learning requires significant computational resources, which may be a barrier for smaller educational institutions with limited budgets. The technical expertise needed to develop and maintain these models can also be a constraint.
Finally, ethical concerns arise from the potential for bias in the data. If the underlying data reflects existing inequalities, unsupervised learning algorithms may reinforce these biases, leading to unfair outcomes for certain student groups.
Addressing these challenges requires careful consideration, robust data handling practices, and ongoing evaluation to ensure that unsupervised learning is applied effectively and equitably in educational settings.
7. Future Trends in Unsupervised Learning for Education
The future of unsupervised learning in education holds exciting possibilities as advancements in technology and data science continue to evolve. One significant trend is the integration of unsupervised learning with artificial intelligence (AI) and machine learning to create more sophisticated educational tools. These tools will be able to offer deeper insights into student learning patterns, allowing for even more personalized and adaptive learning experiences.
Another trend is the increasing use of real-time data analytics. As educational institutions adopt more digital platforms, unsupervised learning algorithms will be able to analyze data in real time, providing immediate feedback and support to students and educators. This will enhance the ability to address learning challenges as they arise, rather than relying on retrospective analysis.
Furthermore, the development of more intuitive and user-friendly unsupervised learning tools will make it easier for educators to interpret and apply data insights without needing advanced technical expertise. This democratization of technology will enable a broader range of educational institutions to benefit from unsupervised learning techniques.
Overall, these trends will drive greater innovation in education, leading to more effective and data-driven approaches to t
8. Conclusion and Summary
Unsupervised learning holds immense potential to revolutionize education by providing educators with potent tools to analyze and interpret complex data, even without pre-existing labels. By leveraging techniques like clustering, association rule mining, and dimensionality reduction, educators can extract valuable insights into student performance, learning styles, and resource utilization. These insights empower educators to personalize learning experiences, optimize curriculum development, and allocate resources efficiently, ultimately leading to improved educational outcomes.
Unsupervised learning holds immense potential, but it faces challenges such as complex data, model interpretability, and resource demands. Overcoming these hurdles is critical for successful implementation and equitable use. Emerging trends point towards integrating unsupervised learning with cutting-edge AI technologies, real-time data analytics, and user-friendly interfaces. This advancement will enhance its capabilities and accessibility, driving innovation in education and facilitating more adaptive and responsive teaching approaches.
Unsupervised learning holds immense promise for education, offering the potential for enhanced personalization, resource optimization, and timely intervention. By addressing current challenges and embracing emerging advancements, educators and institutions can unlock the full power of unsupervised learning, fostering data-driven educational environments that are more effective than ever before. Continued exploration and application of these techniques will pave the way for…
electrapk.com