In the rapidly evolving landscape of education, machine learning is emerging as a powerful tool that can transform teaching and learning experiences. This article explores the integration of machine learning concepts into educational settings, highlighting essential study materials and effective teaching methods. By examining successful case studies and evaluating the impact on student learning outcomes, educators can gain valuable insights into how to harness the potential of machine learning. As we unlock the possibilities of this technology, we pave the way for innovative approaches that enhance educational practices and prepare students for a data-driven future.
Join electrapk.com as we uncover the details of this topic.
1. Introduction to Machine Learning in Education
Machine learning, a branch of artificial intelligence, has transformed numerous industries, and its influence on education is growing rapidly. By harnessing algorithms that can learn from data, educators can tailor learning experiences, refine curriculum development, and boost student involvement. In today’s data-driven society, comprehending machine learning is essential for both educators and students.
Integrating machine learning into education offers numerous benefits. Adaptive learning platforms, powered by machine learning, tailor content to individual student needs, fostering a more personalized learning experience. Additionally, machine learning tools analyze vast amounts of student data, revealing learning patterns and areas where students might struggle. This data-driven insight enables educators to implement timely interventions and support strategies, ultimately leading to improved student outcomes.
In today’s technologically driven educational landscape, the integration of machine learning into curricula has become indispensable. By equipping students with a strong understanding of machine learning concepts and data literacy, we empower them to thrive in the future workforce, which is increasingly reliant on automation and data analysis. This article will explore essential study materials and effective teaching strategies that can guide educators in successfully incorporating machine learning into their classrooms.

2. Key Study Materials for Machine Learning
Teaching machine learning effectively in educational settings requires access to a diverse range of study materials that accommodate various learning styles and levels. A crucial component of these materials is introductory textbooks that thoroughly explain fundamental concepts, algorithms, and applications of machine learning. For instance, the book “Hands-On Machine Learning with Scikit-Learn, Keras, and TensorFlow” offers valuable practical knowledge and hands-on projects for learners.
Online platforms like Coursera and edX provide specialized courses that incorporate video lectures, quizzes, and interactive assignments, allowing students to learn complex subjects at their own pace. Moreover, open-source tools such as Google Colab enable educators and students to experiment with machine learning models in a user-friendly environment, fostering active learning.
Kaggle datasets offer real-world data, providing students with practical opportunities to hone their skills. Tutorials and blogs offer clear, step-by-step explanations that break down complex concepts. Moreover, incorporating case studies showcasing successful machine learning applications across diverse fields can deepen comprehension and inspire students.
By assembling a variety of learning resources, educators can craft a robust learning experience that goes beyond basic knowledge acquisition. Such an experience cultivates critical thinking and problem-solving abilities, crucial for success in the dynamic field of machine learning.
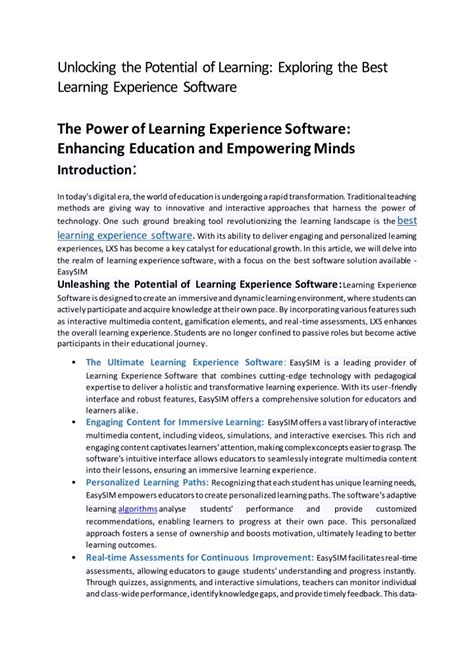
3. Effective Teaching Methods for Machine Learning Concepts
Effective teaching methods for machine learning concepts are crucial for fostering understanding and engagement among students. One prominent approach is project-based learning, where students tackle real-world problems that demand machine learning solutions. This hands-on experience not only reinforces theoretical knowledge but also cultivates critical thinking and problem-solving abilities.
Collaborative learning strategies enhance the educational experience by fostering student interaction. Group work encourages idea sharing, collaborative problem-solving, and the exchange of diverse perspectives. Online discussion forums and collaborative tools further facilitate this interaction, even in remote learning environments.
Flipped classrooms are an effective teaching method that reverses traditional roles. Students access lecture materials outside of class, freeing up classroom time for discussions and hands-on activities. This approach encourages active learning and allows educators to provide individualized support during practical applications.
Multimedia resources like videos, animations, and interactive simulations can make complex machine learning concepts easier to understand. By employing a variety of teaching methods, educators can reach learners with different learning styles, ensuring everyone has the chance to grasp the fundamental principles of machine learning effectively.

4. Case Studies: Successful Integration of Machine Learning in Curricula
Successful integration of machine learning into educational curricula offers valuable insights into best practices and innovative approaches. One noteworthy example is a high school in California that integrated machine learning into its computer science program. Students engaged in projects that involved constructing predictive models using real-world datasets, such as climate data and social media trends. This hands-on experience not only enhanced their technical skills but also sparked interest in data science careers.
A successful example of this approach is evident in a university-level course centered on healthcare analytics. Within this program, students employed machine learning algorithms to analyze patient data, with the objective of enhancing diagnostic accuracy and refining treatment recommendations. Through collaboration with local healthcare providers, students acquired hands-on experience while concurrently contributing to impactful solutions within the healthcare domain.
Furthermore, an online learning platform achieved success by incorporating machine learning modules into its educational program. This innovative approach allowed learners from all corners of the globe to engage with interactive materials and undertake data-driven projects. This model underscores the capacity of online education to make machine learning resources accessible to a wider audience.
These case studies highlight the significant changes that occur when machine learning is incorporated into education. They showcase how practical projects, real-world applications, and collaboration equip students to tackle the challenges of a future driven by data.
5. Evaluating the Impact of Machine Learning on Student Learning Outcomes
To understand the true value of machine learning in education, it’s vital to measure its impact on student learning outcomes. One crucial indicator is student engagement, which can be gauged through surveys and participation in machine learning projects. Schools that have embraced this technology often observe increased student motivation, as the practical applications of machine learning resonate with their interests.
Academic performance serves as a crucial indicator of the program’s effectiveness. Comparing test scores and project grades before and after the introduction of machine learning concepts allows educators to assess the enhancement of students’ comprehension and abilities. Furthermore, monitoring the development of students’ problem-solving skills and data analysis capabilities provides valuable insights into their cognitive growth.
Qualitative feedback from both students and teachers is essential for evaluation. Testimonials and reflections provide valuable insights into personal growth, enhanced critical thinking skills, and increased confidence in handling data-driven tasks.
By combining quantitative data and qualitative insights, we can create a comprehensive evaluation approach that provides a clearer understanding of how machine learning impacts student learning outcomes. This understanding will empower educators to refine their teaching methods and curriculum design, ultimately maximizing their effectiveness.
Incorporating machine learning into education transforms teaching and learning experiences. By utilizing key study materials, effective teaching methods, and real-world applications, educators can engage students and enhance their understanding. Evaluating the impact of these integrations further informs best practices, ensuring that students are well-prepared for a future driven by data and technology.
electrapk.com