From predictive text on our smartphones to self-driving cars, machine learning has become an integral part of our daily lives. This ubiquitous technology, with its potential to revolutionize industries and solve complex problems, is constantly evolving and expanding. In this comprehensive guide, we will delve into the intricacies of machine learning systems, starting with the fundamentals and moving onto the real-world applications and challenges.
Introduction to Machine Learning Systems
At its core, machine learning is a branch of artificial intelligence (AI) that empowers computers to learn from data and improve their performance on a specific task without explicit programming. Instead of being programmed with a set of rules, these systems learn patterns and relationships from data, enabling them to make predictions and decisions. This ability to learn and adapt without human intervention is what sets machine learning systems apart from traditional software.
Key Characteristics of Machine Learning Systems:
- Data-driven: ML algorithms rely heavily on data. The quality and quantity of data directly affect the accuracy and performance of the system.
- Adaptive: Unlike traditional software, ML systems are designed to adapt and improve their performance based on new data.
- Automated: ML systems can automate tasks that would traditionally require human intervention, such as image recognition or fraud detection.
To better understand how machine learning works, let’s take a look at the building blocks of a typical machine learning system.
Understanding the Building Blocks
A typical machine learning system comprises several key components:
- Data: As mentioned earlier, data is the driving force behind machine learning systems. It is fed into the system to train, test, and evaluate the performance of the algorithms.
- Algorithms: These are mathematical models that learn from the data and make predictions or decisions.
- Parameters: Parameters are the variables within the algorithms that are adjusted during the training process to improve the performance of the system.
- Models: Models are the end result of the training process, and they represent the learned patterns and relationships within the data.
- Inference Engine: Once trained, the inference engine uses the model to make predictions or decisions on new data.
Now that we have a basic understanding of the building blocks, let’s explore some of the current innovations in machine learning.
Current Innovations in Machine Learning

Machine learning has come a long way since its inception in the 1950s. With advancements in technology and computing power, there has been a significant surge in the development and application of machine learning systems. Let’s take a look at some of the latest innovations in this field.
Deep Learning
Deep learning is a subset of machine learning that uses artificial neural networks to learn from complex and large datasets. This approach has gained popularity due to its ability to handle tasks such as image and speech recognition, natural language processing, and autonomous driving. Deep learning algorithms have multiple layers that process the data and extract features, allowing for higher accuracy and efficiency.
Reinforcement Learning
Reinforcement learning involves training a system through trial and error to achieve a specific goal. The system receives rewards for taking the right actions and punishments for taking incorrect ones, enabling it to learn and adapt to its environment. This approach has been successfully applied in areas such as robotics, gaming, and finance.
Transfer Learning
Transfer learning refers to the ability of a system to apply knowledge learned from one task to another related task. This technique has been used to reduce the amount of data required to train a model and improve its performance on new tasks. For example, a model trained on image recognition can be transferred to identify objects in a video.
With these cutting-edge innovations, machine learning is making its mark in various industries and applications.
Applications of Machine Learning Systems
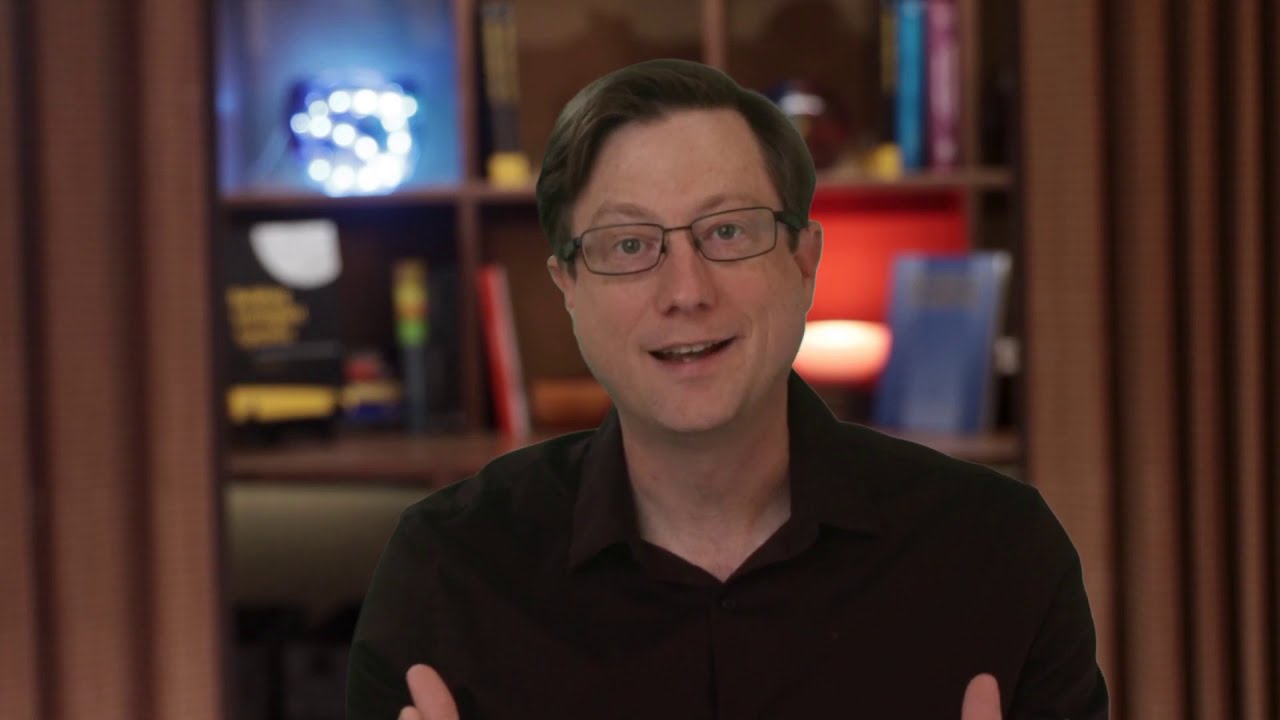
The potential applications of machine learning systems are vast and diverse. From healthcare to finance, and from marketing to transportation, industries across the board are leveraging this technology to improve their processes and outcomes. Let’s take a closer look at some of the popular applications of machine learning.
Healthcare
In the healthcare industry, machine learning is being used to analyze medical data and assist in disease diagnosis and treatment planning. ML systems can analyze patient data and identify patterns that may lead to early detection of diseases or personalized treatment plans. It has also been applied in drug discovery, helping researchers sift through vast amounts of data to identify potential drug candidates.
Finance
Machine learning is transforming the finance industry by automating tasks such as fraud detection, risk assessment, and investment strategies. With the ability to process large amounts of data in real-time, ML systems can quickly identify anomalies and potential risks, enabling financial institutions to make more informed decisions.
Marketing
In the digital age, companies are constantly collecting massive amounts of data on consumer behavior. Machine learning systems can analyze this data and provide insights into customer preferences, trends, and purchasing patterns. This information can then be used to create personalized marketing campaigns and improve customer experience.
Transportation
One of the most exciting applications of machine learning is in the transportation industry, particularly with the development of autonomous vehicles. ML systems can analyze sensor data in real-time and make decisions such as steering, braking, and acceleration, making self-driving cars a reality.
These are just a few examples of how machine learning is being applied in various industries. However, like any other technology, there are challenges and limitations in the field of machine learning.
Challenges and Limitations in the Field
Despite its potential, machine learning still faces some challenges and limitations that need to be addressed for its further advancement and adoption.
Bias in Data
ML systems learn from the data they are fed, and if the data is biased, it can result in biased outcomes. This can lead to discrimination and unfair decisions, particularly in areas such as criminal justice and hiring processes. Developers and researchers need to be aware of this and take steps to address bias in their data and algorithms.
Interpretability
The complex nature of ML algorithms makes it difficult to understand and interpret the decisions made by the system. This lack of interpretability can be a significant challenge, especially in critical applications such as healthcare. Researchers are working towards developing methods to explain the reasoning behind the decisions made by ML systems.
Data Privacy and Security
With the vast amount of data being collected and used for machine learning, privacy and security become major concerns. Sensitive data such as medical records and financial information need to be protected from unauthorized access and potential breaches. Stricter regulations and protocols need to be put in place to ensure the security and privacy of data.
While these challenges and limitations are significant, they do not diminish the potential and importance of machine learning. In fact, with ongoing research and advancements, we are likely to see even more exciting innovations in the future.
Future Trends in Machine Learning Systems
The field of machine learning is constantly evolving, and it is safe to say that there are many more developments and trends yet to come. Here are some of the future predictions and trends in machine learning systems:
- Explainable AI: As mentioned earlier, interpretability is a crucial aspect of machine learning. In the future, we can expect to see more efforts towards making AI more explainable, enabling us to understand and trust the decisions made by these systems.
- Automated Machine Learning (AutoML): AutoML refers to the automation of various steps involved in the machine learning process, such as data preprocessing, feature selection, and algorithm selection. This will make it easier for non-experts to develop and deploy ML systems.
- Edge Computing: With the increasing use of Internet of Things (IoT) devices, there is a growing need for machine learning systems to operate at the edge, i.e., on the device itself rather than in the cloud. This will reduce latency and improve efficiency, making it possible to run ML systems on low-powered devices.
- Federated Learning: Federated learning involves training a model across multiple decentralized devices without sharing their data with a central server. This approach addresses data privacy concerns and allows for faster training and better performance.
These are just a few of the many future trends we can expect to see in the field of machine learning. As technology continues to evolve, we will witness even more exciting developments and applications of this powerful technology.
Conclusion and Summary
In conclusion, machine learning systems have transformed the way we live and work, and their impact is only going to increase in the future. With its ability to learn from data, automate tasks, and make predictions and decisions, ML has the potential to revolutionize industries and solve complex problems.
In this comprehensive guide, we explored the fundamental concepts of machine learning, including its key characteristics and building blocks. We then delved into some of the latest innovations and popular applications of machine learning systems, along with the challenges and limitations faced by this technology. Lastly, we looked at some of the future trends and predictions, giving us a glimpse into what the future holds for machine learning.
As we continue to push the boundaries of technology, machine learning will undoubtedly play a vital role in shaping our world and driving innovation. It is an exciting time to be a part of this constantly evolving field, and the possibilities are endless.